Getting started¶
This notebook showcases the main functionalities of tri3d. It can be run for any of the supported dataset.
[1]:
%matplotlib inline
%load_ext autoreload
%autoreload 2
import k3d
import matplotlib.pyplot as plt
import numpy as np
from tri3d.datasets import KITTIObject, NuScenes, Waymo, ZODFrames
from tri3d.plot import (
plot_bbox_cam,
plot_annotations_bev,
plot_bboxes_3d,
to_k3d_colors,
gen_discrete_cmap,
)
from tri3d.geometry import test_box_in_frame, Rotation
[2]:
# dataset = KITTIObject("datasets/kittiobject")
# camera, image, lidar = "cam", "img2", "velo"
# dataset = NuScenes("datasets/nuscenes", "v1.0-mini")
# camera, image, lidar = "CAM_FRONT", "IMG_FRONT", "LIDAR_TOP"
dataset = Waymo("../../datasets/waymo", split="training")
camera, image, lidar = "CAM_FRONT", "IMG_FRONT", "LIDAR_TOP"
# dataset = ZODFrames(
# "datasets/zodframes",
# "trainval-frames-mini.json",
# "train",
# )
# camera, image, lidar = "front", "img_front", "velodyne"
Pose and calibration¶
In order to plot sensor poses, use Dataset.cam_sensors
and Dataset.pcl_sensors
to get the sensor names. Get the list of available frames for that sensor with Dataset.frames()
. Then use the Dataset.alignment
to get the transformation from that sensor coordinate system to reference axes (in this example we use the lidar at frame 0).
[3]:
plot = k3d.plot(name="pcl", camera_mode="orbit", camera_auto_fit=False)
p0 = dataset.poses(seq=0, sensor=dataset.pcl_sensors[0])[0]
# iterate over sensors
for sensor in dataset.pcl_sensors + dataset.cam_sensors:
if len(dataset.timestamps(seq=0, sensor=sensor)) == 0:
continue
poses = p0.inv() @ dataset.poses(seq=0, sensor=sensor)
# plot axes along the trajectory
origins = poses.apply(np.zeros([3]))
for edge, color in zip(np.eye(3) * 0.2, [0xFF0000, 0x00FF00, 0x0000FF]):
vectors = poses.apply(edge) - origins
plot += k3d.vectors(
origins.astype(np.float32),
vectors.astype(np.float32),
color=color,
head_size=0.3,
group=sensor,
)
plot.camera = [6.44, -4.52, 3.66, 11.35, 6.17, -3.55, 0.0, 0.0, 1.0]
plot
[3]:
Annotations in frame¶
Requesting annotations in image coordinates will automatically interpolate and project annotations to the timestamps and coordinates of the camera.
Two helpers are used to reduce the boilerplate: tri3d.plot.test_box_in_frame
and tri3d.plot.plot_bbox_cam
.
[4]:
sample_image = dataset.image(seq=0, frame=0, sensor=image)
img_size = sample_image.size
sample_annotations = dataset.boxes(seq=0, frame=0, coords=image)
cmap = gen_discrete_cmap(len(dataset.det_labels))
plt.figure(dpi=125, figsize=(10, 6))
plt.xlim((0, img_size[0]))
plt.ylim((img_size[1], 0))
plt.imshow(sample_image)
for i, ann in enumerate(sample_annotations):
# skip boxes where no vertex is in the frame
if test_box_in_frame(ann.transform, ann.size, img_size):
obj_id = str(getattr(ann, "instance", i))[:4]
hdg = ann.heading / np.pi * 180
color = cmap(dataset.det_labels.index(ann.label))
u, v, z = ann.center
print(f" {obj_id:4s}: {ann.label:20s} at {z:3.0f}m heading {hdg:+.0f}°")
# plot the bounding box
plot_bbox_cam(ann.transform, ann.size, img_size, c=color)
# plot the uid
plt.text(
np.clip(u, 0, img_size[0]),
np.clip(v, 0, img_size[1]),
obj_id,
horizontalalignment="center",
verticalalignment="center",
bbox=dict(facecolor="white", linewidth=0, alpha=0.7),
)
0 : VEHICLE at 30m heading -12°
1 : VEHICLE at 42m heading -9°
3 : SIGN at 34m heading -190°
6 : VEHICLE at 20m heading -100°
10 : SIGN at 34m heading -190°
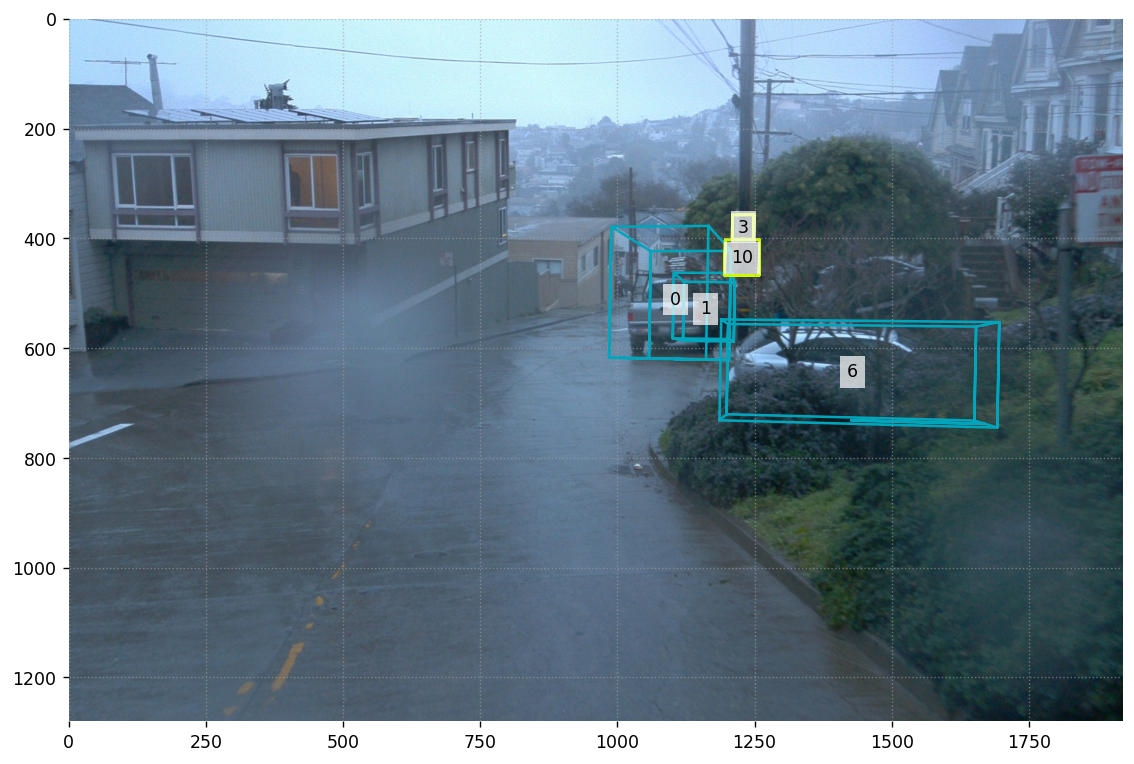
Lidar points over images¶
Requesting points in image coordinates will automatically compensate for ego car movement between the two sensor acquisitions and for extrinsic and intrinsic calibrations.
[5]:
points = dataset.points(seq=0, frame=0, sensor=lidar, coords=image)
# discard extra dims (intensity, etc.)
uvz = points[:, :3]
# only keep visible points
in_frame = (
np.all(uvz > 0, axis=1) # in front of the camera
& (uvz[:, 0] < sample_image.size[0])
& (uvz[:, 1] < sample_image.size[1])
)
uvz = uvz[in_frame]
plt.figure(dpi=125, figsize=(10, 6))
plt.xlim((0, img_size[0]))
plt.ylim((img_size[1], 0))
plt.imshow(sample_image)
plt.scatter(uvz[:, 0], uvz[:, 1], c=uvz[:, 2], clim=(0, 30), s=0.5, alpha=1)
plt.xlim(0, sample_image.size[0])
plt.ylim(sample_image.size[1], 0)
plt.gca().set_aspect("equal")
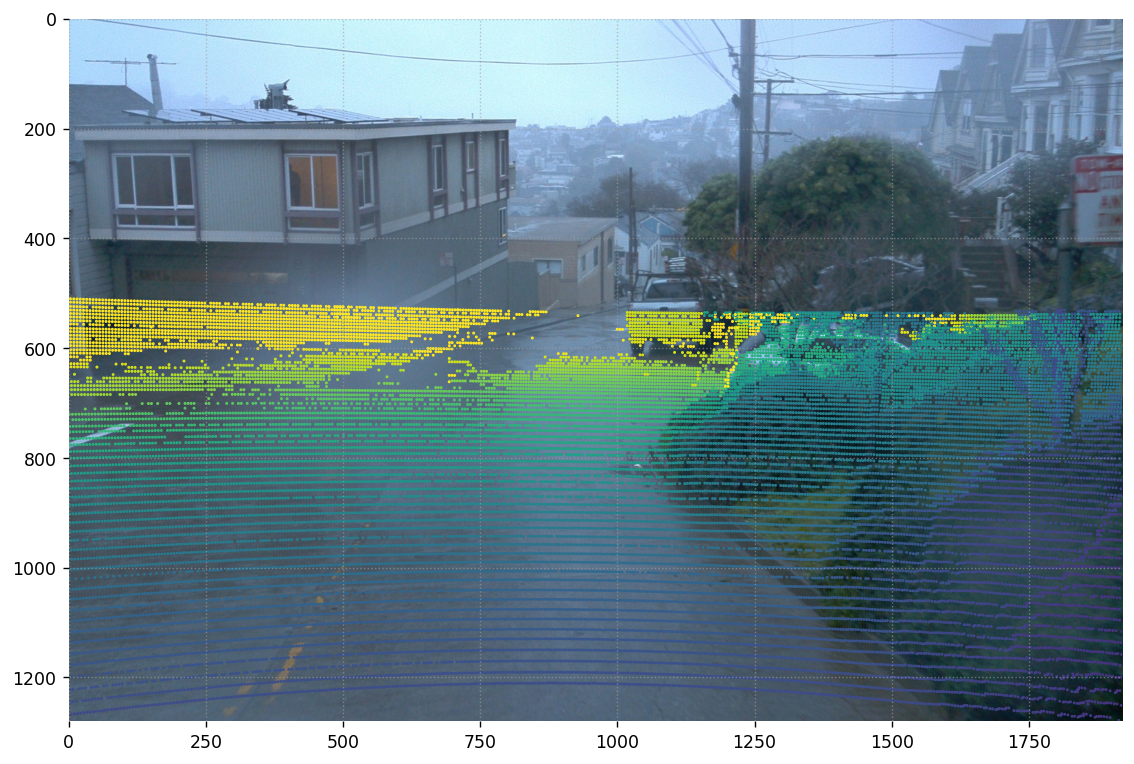
Lidar points and boxes¶
[6]:
sample_pcl = dataset.points(seq=0, frame=0, coords=lidar, sensor=lidar)
sample_annotations = dataset.boxes(seq=0, frame=0, coords=lidar)
plt.figure(dpi=125, figsize=(8, 8))
plt.scatter(
sample_pcl[:, 0], sample_pcl[:, 1], s=5, c="k", alpha=0.6, edgecolors="none"
)
cmap = gen_discrete_cmap(len(dataset.det_labels))
plot_annotations_bev(
[a.center for a in sample_annotations],
[a.size for a in sample_annotations],
[a.heading for a in sample_annotations],
ax=plt.gca(),
c=cmap([dataset.det_labels.index(a.label) for a in sample_annotations]),
)
plt.xlim(-25, 25)
plt.ylim(-25, 25)
plt.gca().set_aspect("equal")
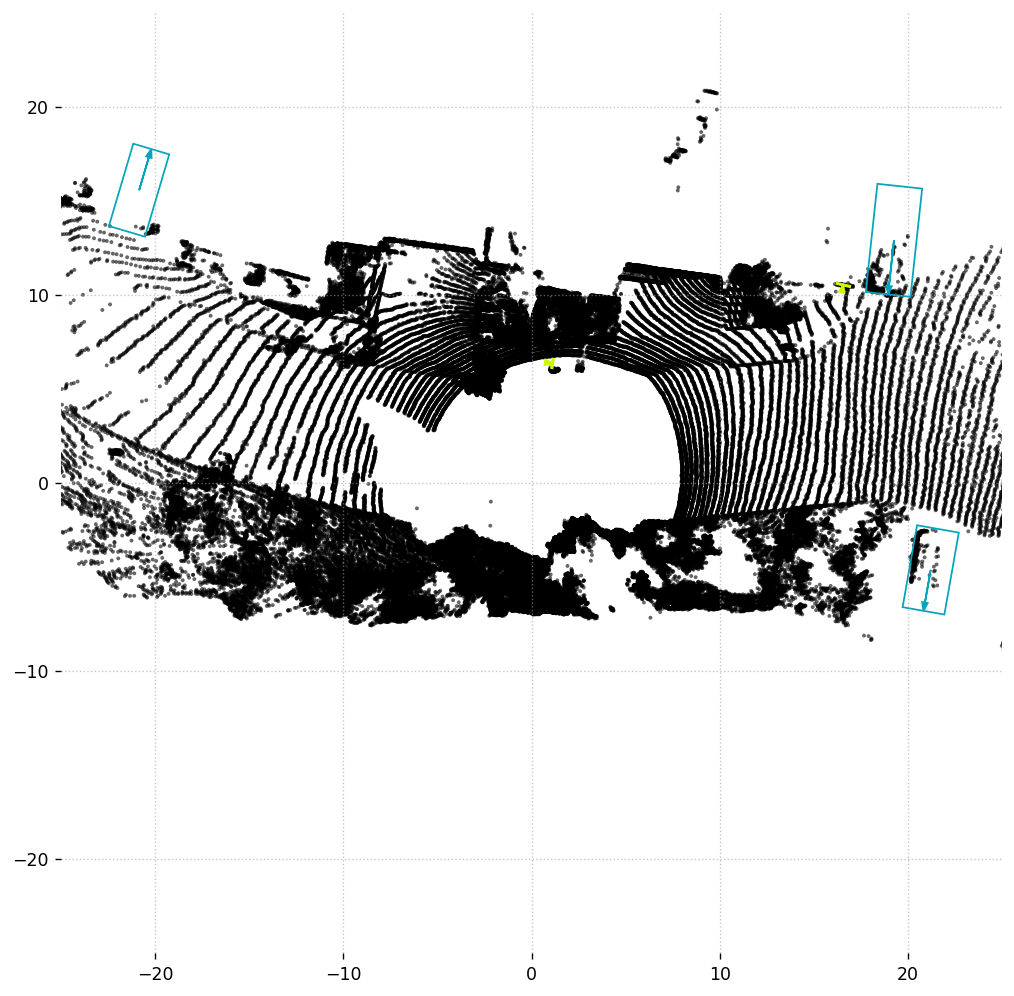
[7]:
xyz = dataset.points(seq=0, frame=0, coords=lidar, sensor=lidar)[:, :3].astype(np.float32)
sample_annotations = dataset.boxes(seq=0, frame=0, coords=lidar)
sample_annotations = [a for a in sample_annotations if a.label != "DontCare"]
c = to_k3d_colors(plt.get_cmap("viridis")((xyz[:, 2] + 2) / 4))
plot = k3d.plot(name="pcl", camera_mode="orbit", camera_auto_fit=False)
plot += k3d.points(positions=xyz, point_size=1, shader="dot", colors=c)
plot_bboxes_3d(
plot,
[a.transform for a in sample_annotations],
[a.size for a in sample_annotations],
c=0xFF0000,
)
plot.camera = [-0.52, -12.19, 17.0, 4.94, -1.7, 5.19, 0.0, 0.0, 1.0]
plot
[7]:
[8]:
# if isinstance(dataset, Waymo): # waymo does not have segmentation for all frames
# seq, frame = dataset.frames(0, sensor="SEG_LIDAR_TOP")[0]
# else:
# seq, frame = 0, 0
# xyz = dataset.points(seq, frame, sensor=lidar)[:, :3]
# semantic = dataset.semantic(seq, frame, sensor=lidar)
# cmap = gen_discrete_cmap(len(dataset.sem_labels))
# c = to_k3d_colors(cmap(semantic)) * (semantic >= 0)
# plot = k3d.plot(name="pcl", camera_mode="orbit")
# plot += k3d.points(positions=xyz, point_size=1, shader="dot", colors=c)
# plot